3D printing is quite effective for building prototypes prior to full-scale manufacturing. However, 3D printers are faster and now able to produce forms in volumes that compete with traditional manufacturing methods..
Let’s look at some of the advantage of 3D printing and additive manufacturing (AM):
- It is quite economical and produces much less waste than traditional manufacturing
- It has become fast, easy, and requires minimal tooling and equipment.
- It can create the widest array of products: from fine printed circuit boards, to a large house.
But is the quality sufficient? Can we safely say that additive manufacturing and 3D printing has the same level of quality as has been established through the years since the introduction of - Eli Whitney's cotton gin and the whole ideas of mass production?
Let’s look at the quality control tools and techniques currently available to ensure that your 3D printed parts are manufactured to spec and perform as required. Then we’ll explore the growing need for and work toward quality standards in additive manufacturing.
It is great to have quality standards and ways additively manufactured products can be compliant. Now, for the AM industry, the question is: how? What techniques and tools do we have at our disposal, and which are particularly relevant for AM processes?
To meet the requirements of any manufactured product, you need data. To get GD&T (Geometrical Dimensioning and Tolerances) measurements, investing in high-precision metrology equipment for your specific purpose is a must. So, let’s look at some of these tool options currently available in the market.
Measurement Precision
Additive manufacturing relies on digital scanning and control of dimensions. This allows for precise fabrication that many industries demand.
With digital tools, very small differences may be used to auto correct any anomalies and make any needed adjustments to the fabrication process. In addition to CMM, optical scanning can be put to work without meeting the finished work.
Using Advanced Mathematics to Assure Quality
Florida Agricultural Mechanical University and Florida State University (FAMU-FSU College) were jointly awarded a National Science Foundation (NSF) grant to study quality issues 3D printing. FAMU-FSU College of Engineering developed a new technology enabling different printers to share learned experiences and lessons with each other. The process shows much promise as a boost to 3-D printing system efficiency.
In an article on the school's website, one of the researchers, Hui Wang, was quoted as saying “Cloud manufacturing, along with the Internet of Things (IoT), is a technology that has emerged in recent decades,” said Wang, associate professor at the FAMU-FSU College of Engineering. “The technology demonstrates that data generated from multiple production machines can be shared with each in a timely manner, and manufacturing can be enclosed as online services for meeting diverse market demands.”
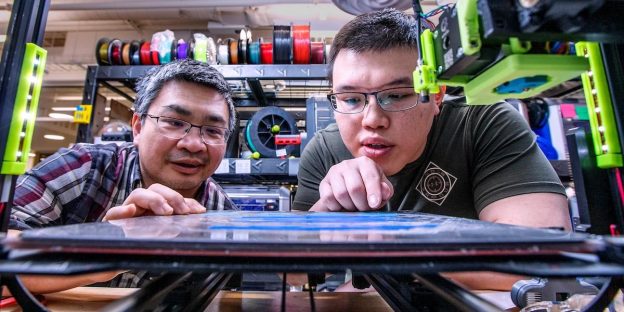
Hui Wang, left, associate professor of industrial engineering and An-Tsun Wei, a Ph.D. student, are the co-authors of a paper detailing how learning cloud data collected from interconnected 3D printers improves quality control and printing accuracy. (M Wallheiser/FAMU-FSU Engineering)
Wang and other researchers are developing novel learning algorithms and process control methodologies that will use cloud data. Ultimately, the algorithms will help curb printing defects and out-of-tolerance issues that may affect quality of printed structures.
Another researcher said that calculating the probabilities and pinpointing any deformities in a print fabrication evolution is a huge step forward as it allows the same precision used to make something, help correct any deviations from quality.
“We crafted a model that helps predict the infill pattern of non-uniformity that occurs,” An-Tsun Wei, a Ph.D. student in IME and co-author of the paper, said. “We can estimate geometric print quality and the related defects that might occur with the model. The information can be used to calculate adjustments needed in the input printing parameters to compensate for those errors.”
Using Machine Learning to Achieve Finite Geometric Accuracy
Purdue University is off with a similar quality calculation using a "closed-loop" system to monitor quality. They realize that geometric inaccuracies and deviations in shape between the printed product and the nominal CAD model is a limiting factor in wider adoption of printed products.
Purdue describes their method as a "closed-loop machine learning and compensation framework that can improve geometric accuracy control of 3D shapes in AM systems." They say that their framework is based on a Bayesian extreme learning machine (BELM) architecture. This leverages data and deviation models from previously printed products to transfer deviation models thus identifying any patterns in deviation.
"The closed-loop nature of compensation under our framework, in which past compensated products that do not adequately meet dimensional specifications are fed into the BELMs to re-learn the deviation model, enables the identification of effective compensation plans and satisfies resource constraints by printing only one new shape at a time," they describe in an article on their website. "The power and cost-effectiveness of our framework are demonstrated with two validation experiments that involve different geometries for a Markforged Metal X AM machine printing 17-4 PH stainless steel products. As demonstrated in our case studies, our framework can reduce shape inaccuracies by 30% to 60% (depending on a shape's geometric complexity) in at most two iterations, with three training shapes and one or two test shapes for a specific geometry involved across the iterations. We also perform an additional validation experiment using a third geometry to establish the capabilities of our framework for prospective shape deviation prediction of 3D shapes that have never been printed before."
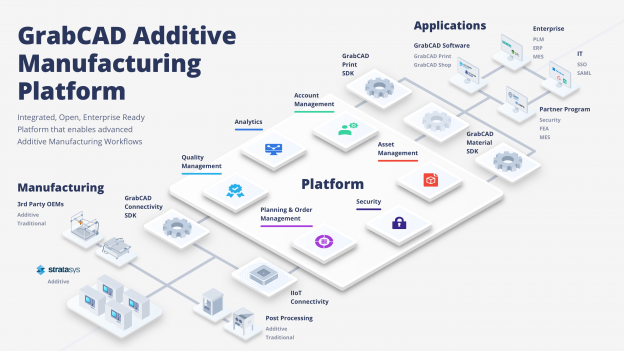
If you would like to learn more about Industry 4.0 and how things like machine learning can impact your additive manufacturing operations like quality managment, GrabCAD offers a free AM evaluation where you can speak to a PM to learn how you can scale and maximize your production.
Quality assurance for the manufacturing of precision parts is still a work in progress. In many ways we’re almost there. When we do, expect the quality of production parts fabricated with 3D printing and AM to be stellar and unprecedented as new technologies and accurate means of achieving quality become the norm.